Today, most practical machine learning models utilize supervised learning, which applies an algorithm to map one input to one output. For supervised learning to work, you need a labeled set of data that the model can learn from to make correct decisions. Vodafone tv sky go. Data labeling typically starts by asking humans to make judgments about a given piece of unlabeled data. Atomic mass of cl. For example, labelers may be asked to tag all the images in a dataset where 'does the photo contain a bird' is true. The tagging can be as rough as a simple yes/no or as granular as identifying the specific pixels in the image associated with the bird. The machine learning model uses human-provided labels to learn the underlying patterns in a process called 'model training.' The result is a trained model that can be used to make predictions on new data.
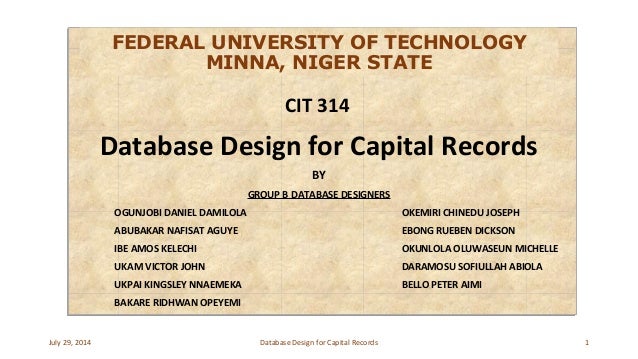
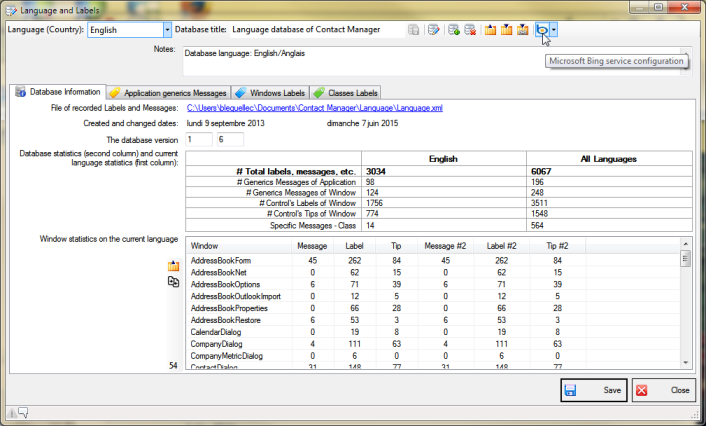
The online Label Search is a flexible tool that is used to gather pesticide product information by searching the available Product Information Elements, or the full textual content of the entire label collection from the Pest Management Regulatory Agency (PMRA) Registered Products Database. Dietary Supplement Label Database (DSLD) The Dietary Supplement Label Database (DSLD) from the National Institutes of Health (NIH) contains information taken from the labels of approximately 76,000 dietary supplement products available in the U.S.
Clothing Label Database

:format(jpeg):mode_rgb():quality(90)/discogs-images/R-14489895-1575584783-2074.jpeg.jpg)
The online Label Search is a flexible tool that is used to gather pesticide product information by searching the available Product Information Elements, or the full textual content of the entire label collection from the Pest Management Regulatory Agency (PMRA) Registered Products Database. Dietary Supplement Label Database (DSLD) The Dietary Supplement Label Database (DSLD) from the National Institutes of Health (NIH) contains information taken from the labels of approximately 76,000 dietary supplement products available in the U.S.
Clothing Label Database
Label Database Software
In machine learning, a properly labeled dataset that you use as the objective standard to train and assess a given model is often called 'ground truth.' The accuracy of your trained model will depend on the accuracy of your ground truth, so spending the time and resources to ensure highly accurate data labeling is essential.